Recognizing Words from Gestures: Discovering Gesture Descriptors Associated with Spoken Utterances
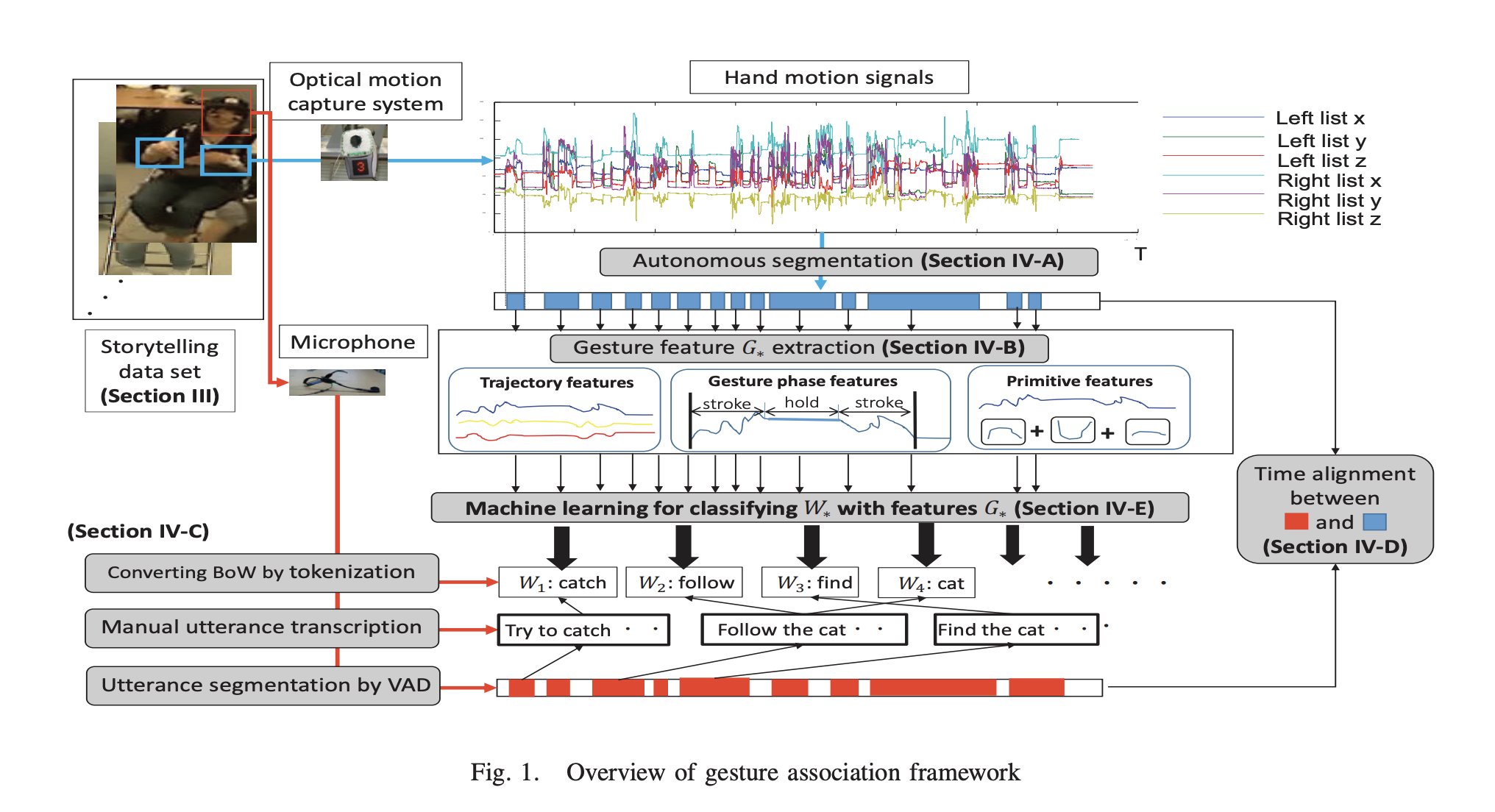
This study investigates a new challenge: modelling the relationship between gestures and spoken words during continuous hand motions, speech and language data. The problem setting and modelling is defined as “gesture association” (associating gestures with words). We present a framework to associate spoken words with hand motion data observed from a optical motion-capture system. The framework identifies pairs of hand motions (gesture) and uttered words as training samples by autonomously aligning the speech and the co-occurring gestures. Using the samples, a supervised learning approach is undertaken to learn a model to discriminate between gestures that co-occur with a spoken word (w) and gestures when the word w is not being spoken. To detect gestures and associate them with spoken words, we extract (1) a trajectory signal feature set, (2) features of various gesture phases from hand motion data and (3) primitive gesture patterns learned by Sift Invariant Sparse Coding (SISC). Then Hidden Markov Model (HMM) and Support Vector Machine (SVM) classifiers were trained with these three-feature sets. In experiments, the classification accuracy achieved for 80 words was above 60 % (the maximum accuracy achieved was 71 %) using the proposed framework. In particular, SVMs trained with features (2) and (3) outperform an HMM trained with feature (1) (prepared as a baseline model). The results show that the gesture phase and primitive patterns trained by SISC are effective gesture features for recognizing the words accompanying gestures.
full version link