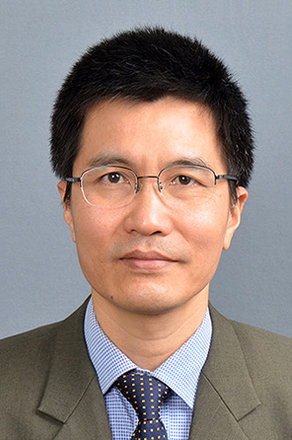
Decision Intelligence
Laboratory on Decision Intelligence
Professor:HUYNH, Nam Van
E-mail:
[Research areas]
Data Science, Artificial Intelligence, Operations Research, Decision Support Systems
[Keywords]
Machine learning, Data analytics, Argumentation, Optimization, Uncertainty management, Decision analysis
Skills and background we are looking for in prospective students
Our lab gladly welcomes highly motivated students who have acquired a solid background and skills through their undergraduate/graduate program, and who have a willingness to study actively and cooperatively.
What you can expect to learn in this laboratory
Students are expected to develop valuable knowledge and problem-solving skills that can be applied in the areas of practical applications of data science and decision analysis. These problem-solving skills include the ability of 1) identifying and formulating decision problems; 2) acquiring and modelling knowledge for solving them; and 3) generating, evaluating, validating and implementing solutions to these problems.
【Job category of graduates】 Academic jobs in universities, IT industry, Consulting and marketing companies, etc.
Research outline
With the research vision that Data Science paired with (data-driven) Knowledge Management will provide the core of Intelligent Decision-Support Systems, the strategic mission of our laboratory is to develop both fundamental research and applied research relating to the creation, integration, reasoning and use of knowledge from data in intelligent decision support systems.
In particular, our current research interests lie in data analytics and machine learning (ML), AI reasoning, uncertainty management, operations research and decision making. The synergy of these research areas will allow us to provide adaptivity at all levels of intelligent decision support systems in today’s era of big data, from acquiring, fusing and reasoning to using knowledge for supporting decision-making.
As graphically illustrated in the figure, we explore fundamental topics in these research areas combined with practical applications in fields ranging from e-commerce and marketing intelligence to finance, industrial and business management. Such an approach essentially helps to guide theoretical research, ensuring that it is relevant and significant, while providing novel and advanced solutions to practical problems and research questions. Some specific topics are as follows:
Key publications
- D.-V. Vo, J. Karnjana, V.-N. Huynh. An integrated framework of learning and evidential reasoning for user profiling using short texts, Information Fusion 70 (2021), 27-42.
- D.-H. Nguyen, V.-N. Huynh. Revealed preference in argumentation: Algorithms and applications. Intern. J. of Approximate Reasoning 131 (2021), 214-251.
- T. Nguyen-Mau, V.-N. Huynh. An LSH-based k-representatives clustering method for large categorical data. Neurocomputing 463 (2021), 29-44.
- V.-D. Nguyen, V.-N. Huynh, S. Sriboonchitta. Integrating community context information into a reliably weighted collaborative filtering system using soft ratings. IEEE Transactions on Systems, Man, and Cybernetics: Systems 50 (2020), 1318-1330.
Teaching policy
The guiding principle of research in the laboratory is that a well-developed decision support system must be based on a sound theoretical foundation. Our education and research strategy is therefore trying to balance the advancement of theoretical research and its applicability in practice. Students will be exposed to relevant practical problems during development and improvement of their systems thinking and modelling competencies, which in turn helping them get insights into problems, inspired and guided theoretical research (making it relevant, innovative, effective and significant) in knowledge modelling and decision making. This strategy is done through regularly-held lab meetings and activites.
[Website] URL:https://www.jaist.ac.jp/~huynh/