Estimating interviewee's willingness in multimodal human robot interview interaction
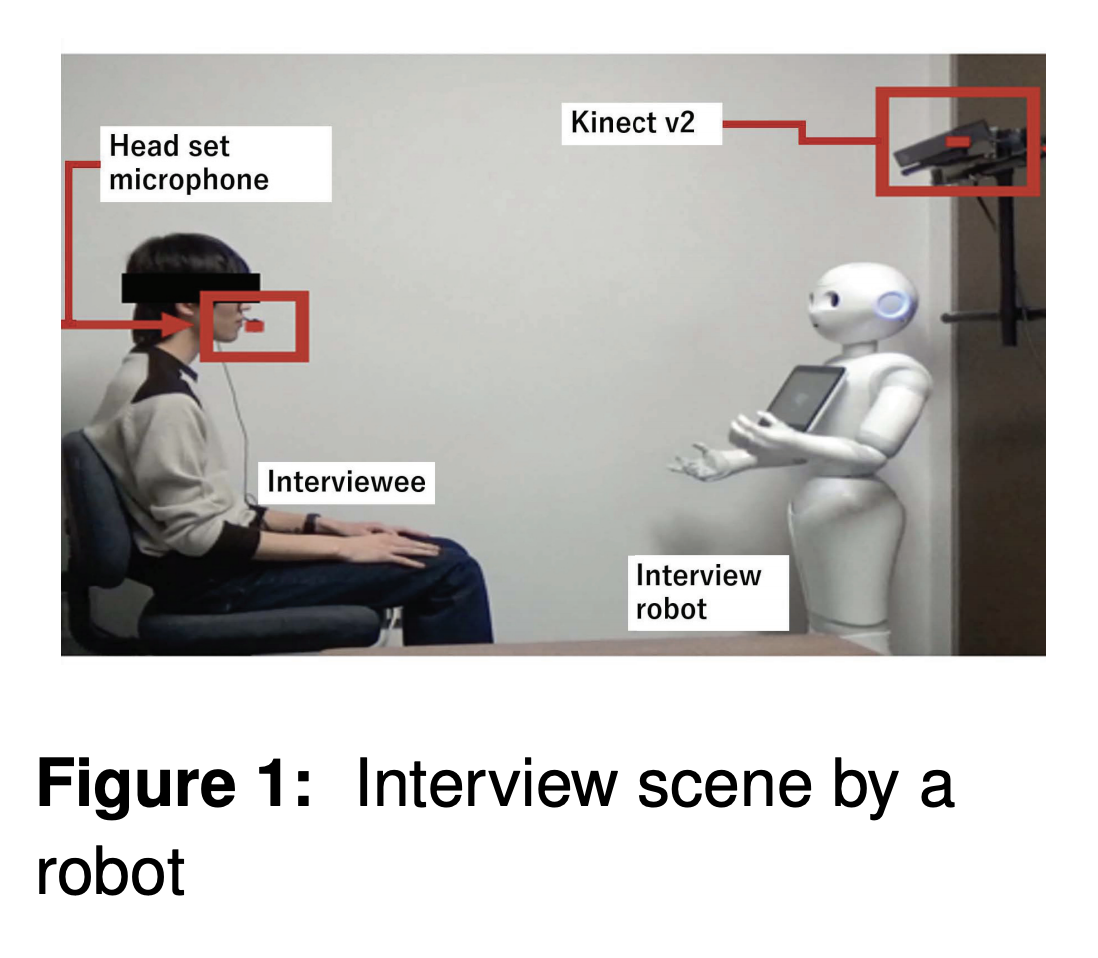

This study presents a prediction model of a speaker's willingness level in human-robot interview interaction by using multimodal features (i.e., verbal, audio, and visual). We collected a novel multimodal interaction corpus, including two types of annotation data sets of willingness. A binary classification task of the willingness level (high or low) was implemented to evaluate the proposed multimodal prediction model. We obtained the best classification accuracy (i.e., 0.6) using the random forest model with audio and motion features. The difference between best accuracy (i.e., 0.6) and coder's recognition accuracy (i.e., 0.73) was 0.13.
full version link