Dementia Scale Classification Based on Ubiquitous Daily Activity and Interaction Sensing
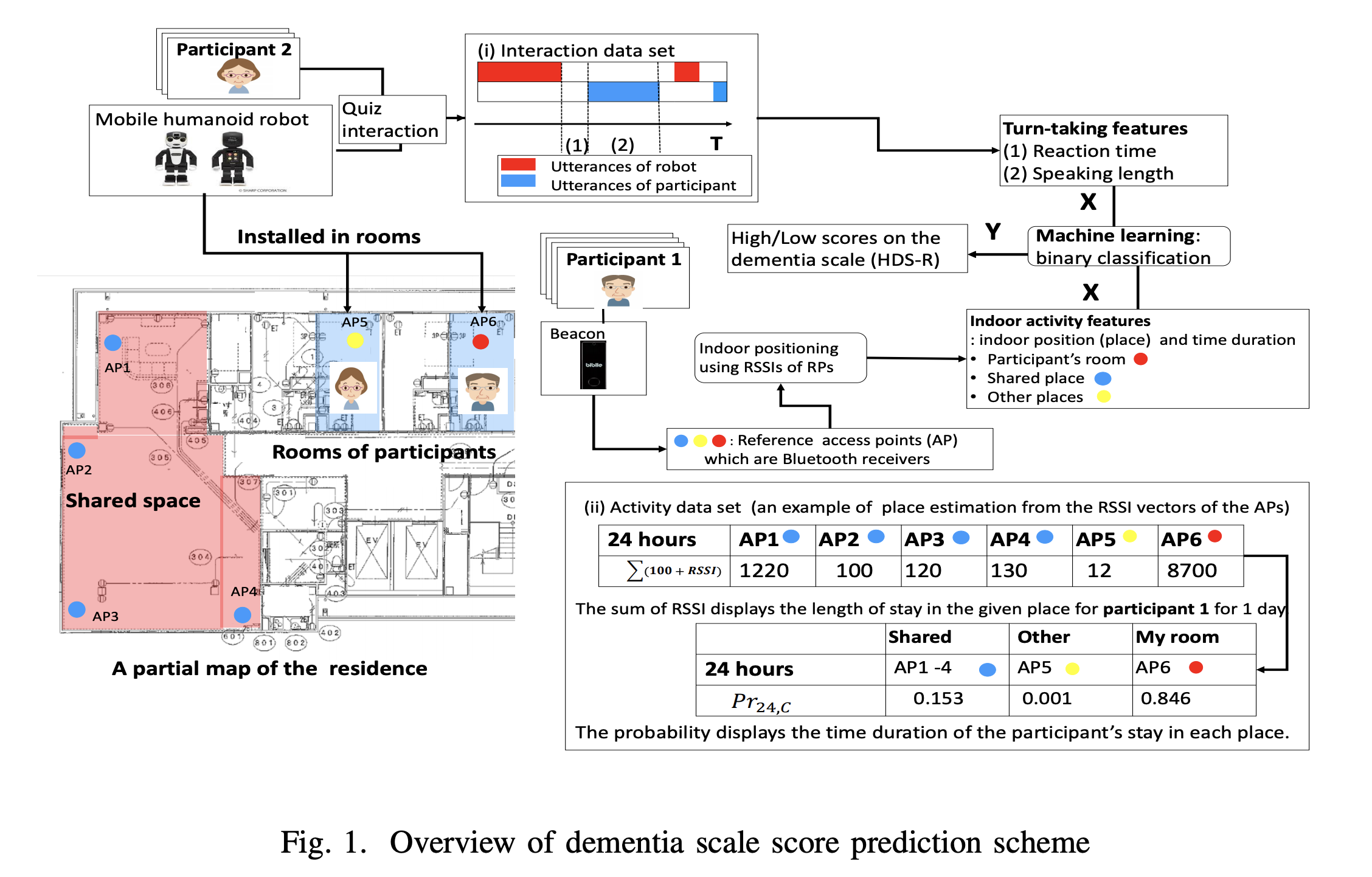
This paper investigates the integration of differentapproaches to automatically predict high/low-score on the de-mentia scale.
We propose two different approaches to predict thisvalue by capturing the following: (1) the participant’s interactionbehavior with a humanoid robot and (2) the indoor dailyactivity in the residence using ubiquitous sensors. The interactionand indoor activity data set were obtained by recording 32participants living in common residences, including 17 withsymptoms of dementia, as indicated through a cognitive test(Revised Hasegawa Dementia Scale). To obtain the interactionfeatures, we extracted the turn-taking features of interaction witha mobile-typed humanoid robot. To extract the indoor activityfeatures, we collected the location data of each participant in theresidence using the received signal strength indicators (RSSIs) ofBluetooth signals from different access points (e.g., shared spacesor the participant’s room). In the experimental evaluation, wetrained binary classification models for classifying the score onthe dementia scale from these datasets. The results show that thebest classification accuracy (0.875) is achieved when interactionand activity features are fused using a random forest classifier.Index Terms—Dementia, Ubiquitous indoor positioning, Hu-man robot interaction, Machine learning
full version link