Associate Professor Shogo Okada, Intelligent Robotics Area, received Two Best Paper Runner-up Awards at the 21st ACM International Conference on Multimodal Interaction (ICMI 2019)
Associate Professor Shogo Okada, Intelligent Robotics Area, received Two Best Paper Runner-up Awards at the 21st ACM International Conference on Multimodal Interaction (ICMI 2019).
This conference was held in Suzhou, China on October 14 through 18, 2019. The focus of this conference is on the technical and practical aspects of the multimodal interaction.
They proposed a paper "Secure Online-Efficient Interval Test Based on Empty-Set Check" and have achieved a secure online-efficient interval test by adopting the notion of online and offline phases.
■Date Awarded
October 17, 2019
■Information of papers
- Title:
Multitask Prediction of Exchange-Level Annotations for Multimodal Dialogue Systems
Authors:
Yuki Hirano (JAIST), Shogo Okada (JAIST), Haruto Nishimoto (Osaka University), Kazunori Komatani (Osaka University)
Abstract:
This paper presents multimodal computational modeling of three labels that are independently annotated per exchange to implement an adaptation mechanism of dialogue strategy in spoken dialogue systems based on recognizing user sentiment by multimodal signal processing. The three labels include (1) user's interest label pertaining to the current topic, (2) user's sentiment label, and (3) topic continuance denoting whether the system should continue the current topic or change it. Predicting the three types of labels that capture different aspects of the user's sentiment level and the system's next action contribute to adopting a dialogue strategy based on the user's sentiment. For this purpose, we enhanced shared multimodal dialogue data by annotating impressed sentiment labels and the topic continuance labels. With the corpus, we develop a multimodal prediction model for the three labels. A multitask learning technique is applied for binary classification tasks of the three labels considering the partial similarities among them. - Title:
Task-independent Multimodal Prediction of Group Performance Based on Product Dimensions
Authors:
Go Miura (JAIST), Shogo Okada (JAIST)
Abstract:
This paper proposes an approach to develop models for predicting the performance for multiple group meeting tasks, where the model has no clear correct answer. This paper adopts "product dimensions" [Hackman et al. 1967] (PD) which is proposed as a set of dimensions for describing the general properties of written passages that are generated by a group, as a metric measuring group output. This study enhanced the group discussion corpus called the MATRICS corpus including multiple discussion sessions by annotating the performance metric of PD. We extract group-level linguistic features including vocabulary level features using a word embedding technique, topic segmentation techniques, and functional features with dialog act and parts of speech on the word level. We also extracted nonverbal features from the speech turn, prosody, and head movement. With a corpus including multiple discussion data and an annotation of the group performance, we conduct two types of experiments thorough regression modeling to predict the PD.
■Comment
It is a great honor to receive Two Best Paper Runner-up Awards at the 21st ACM International Conference on Multimodal Interaction (ICMI 2019).
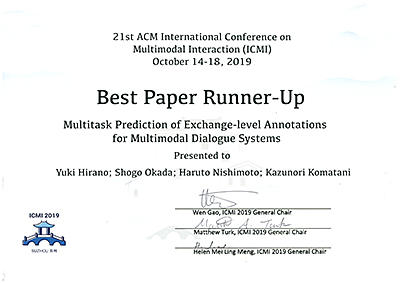
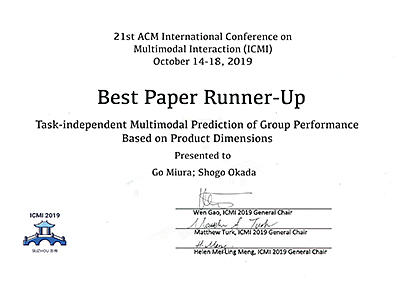
November 1, 2019